The Applications of AI in the Current Healthcare System - Part One
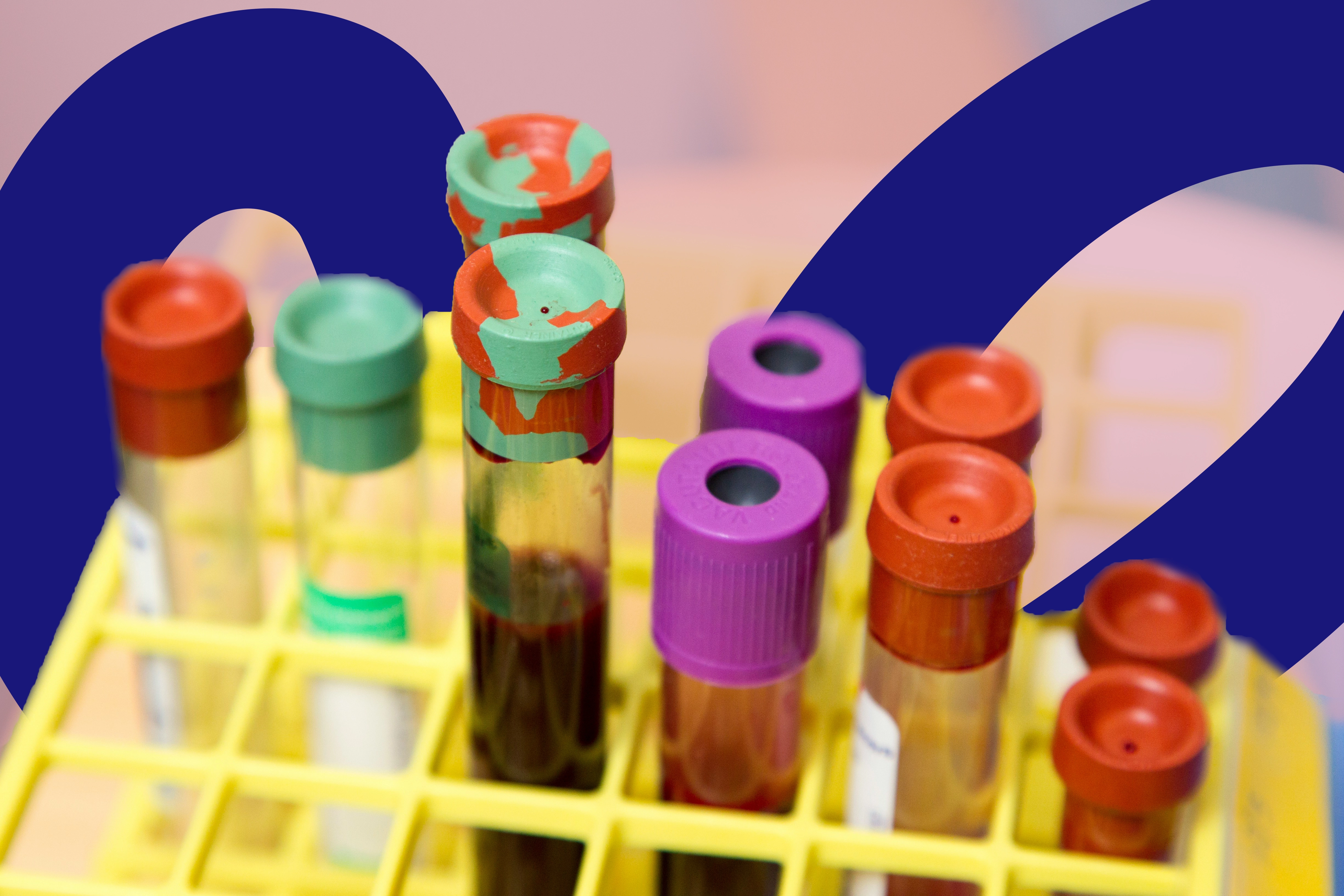
This is in continuation to the previous blog, we have seen what role AI plays in healthcare. We have identified three areas -
- AI-led drug discovery,
- Clinical trials, and
- Patient Care. (This will be discussed next week)
In this blog we are going to see how AI is being used in these areas together termed as Medical AI.
- AI for drug discovery
AI technology in healthcare has helped pharmaceutical companies speed up their drug discovery process. AI is used here to automate the identification of targets. In addition, by analyzing off-target compounds, AI is also aiding in drug repurposing. As a result, AI is able to streamline the concurrent process and streamline drug discovery.
Several remedies discovered by the leading biopharmaceutical companies are available. Pfizer is utilizing IBM Watson, a machine learning-based system to help finding immune-oncology treatments. Sanofi has agreed to employ Exscientia’s artificial intelligence platform to design metabolic disease medications, while Roche subsidiary Genentech is relying on a AI system from gas healthcare in Cambridge, Massachusetts, to aid in its search for cancer treatments. Almost every major biopharmaceutical company has comparable collaborations or internal programs.
If proponents of these strategies are correct, AI and machine learning will bring in a new era of drug development that is faster, cheaper, and more effective. Some are skeptical, but most experts believe these tools will become more crucial in the future. Scientists face both obstacles and opportunities as a result of this transformation, particularly when the approaches are coupled with automation.
- AI for clinical trials
A clinical trial is a procedure in which freshly manufactured treatments are given to people to test how well they work. This has taken a significant amount of time and money. The success rate, however, is quite low. As a result, clinical trial automation has proven to be a benefit for the application of AI in the healthcare business. Furthermore, the application of AI in healthcare assists in the elimination of time-consuming data monitoring procedures. Additionally, AI-assisted clinical trials handle large amounts of data and produce very accurate outcomes. The following are some of the most popular AI in healthcare applications for clinical trials.
- Intelligence clinical trials
Traditional ‘linear and sequential’ clinical trials are still the gold standard for ensuring the efficacy and safety of new drugs. The lengthy, tried-and-true method of distinct and defined stages of Randomized Controlled Trials (RCTs) was developed primarily for evaluating mass market for pharmaceuticals and has remained mostly unchanged in recent decades. Artificial intelligence has the potential to shorten clinical trial cycle durations while also enhancing productivity and clinical development outcomes. This is the third in a series of reports on AI’s impact on the biopharma value chain. Biopharma businesses have been able to obtain increasing volumes of scientific and research information from a multitude of sources in recent years, which is referred to as real-world data (RWD). They have, however, frequently lacked the expertise and tools needed to successfully use this data. Applying predictive AI models and advanced analytics to unlock RWD can help researchers better understand diseases, find relevant patients and important investigators, and enable revolutionary clinical study designs.
In combination with an efficient digital infrastructure, clinical trials data might be cleansed, aggregated, coded, preserved, and maintained using AI algorithms. Furthermore, improved Electronic Data Capture (EDC) may reduce the impact of human error in data collection while also allowing for smooth system integration.
- Clinical trial cooperation and model sharing
Researchers from several domains are racing to support the reaction to COVID-19 in an exceptional effort of scientific collaboration. Making a worldwide effect with AI tools would necessitate scalable data, models and code sharing strategies, as well as application adaptation to local settings and cross-border collaboration.
Data is required for AI applications. There are presently dozens of data-sharing efforts centred on COVID-19, spanning the worldwide, national, and local levels. Genetic sequences, genomic analyses, protein structures, patients clinical information, medical imagery, event data, epidemiological information, movement data, social media comments, news stories, and scientific literature are just a few of the resources available. Hyper fragmentation of data-sharing activities is a problem since it could lead to advancements that are limited to only specific projects and communities. The creation and diffusion of new applications could be accelerated by establishing scalable techniques for data, model, and code sharing. Global, open, comprehensive, comparable, and verifiable data-sharing activities will be useful at this stage in connecting and promoting cooperation between various communities and geographies.
Open science, aided by multi-stakeholder AI collaborations that operate across international borders, can speed up information distribution and capacity building in national health systems. The Epidemic Intelligence form Open Source (EIOS) network, for example, uses open source data to enable early detection, verification, and assessment of public health hazards and threats.
The network of practice for healthcare intelligence comprises governments, international organisations, and research institutions that collaborate to assess and share information covering outbreak occurrences in real time under the principle of collaboration rather than competition in early detection. Global standards and databases interoperability may facilitate unified reaction and decision-making at the global, national, and local levels, according to epidemiologists. Understanding the epidemiological characteristics and risk features of various demographics as the pandemic progresses will necessitate taking into account healthcare resource capacity, public health measures, environmental factors, and societal consequences.
Aside from data sharing, there are currently few projects that exchange trained AI models of any of the suggested uses. Constraints imposed by unique computational, design, and infrastructural needs; a shortage of documenting; verification and interoperability issues; and legal concerns about confidentiality and intellectual property are among the obstacles to be overcome. Sharing pre-trained and approved AI models used to diagnose illness from pictures, forecast patient results, filter misinformation depending on propagating patterns through social media, and distil knowledge graphs from massive collections of scholarly paper are instances of algorithms that could be broadly useful.
References
Agrawal, J. (2018). Stethee, an AI Powered Electronic Stethoscope. Anaesthesia, Pain & Intensive Care, 22(3), 412–413.
Agrawal, P. (2018). Artificial intelligence in drug discovery and development. J Pharmacovigil, 6(2).
Angus, D. C. (2020). Randomized clinical trials of artificial intelligence. Jama, 323(11), 1043–1045.
Chan, H. C. S., Shan, H., Dahoun, T., Vogel, H., & Yuan, S. (2019). Advancing drug discovery via artificial intelligence. Trends in Pharmacological Sciences, 40(8), 592– 604.
Cruciger, O., Schildhauer, T. A., Meindl, R. C., Tegenthoff, M., Schwenkreis, P., Citak, M., & Aach, M. (2016). Impact of locomotion training with a neurologic controlled hybrid assistive limb (HAL) exoskeleton on neuropathic pain and health related quality of life (HRQoL) in chronic SCI: a case study. Disability and Rehabilitation: Assistive Technology, 11(6), 529–534.
Díaz, Ó., Dalton, J. A. R., & Giraldo, J. (2019). Artificial intelligence: a novel approach for drug discovery. Trends in Pharmacological Sciences, 40(8), 550–551.
Greenberg, N., Docherty, M., Gnanapragasam, S., & Wessely, S. (2020). Managing mental health challenges faced by healthcare workers during covid-19 pandemic. Bmj, 368. Habermann, J. (2021). Psychological impacts of COVID-19 and preventive strategies: A review.
Harrer, S., Shah, P., Antony, B., & Hu, J. (2019). Artificial intelligence for clinical trial design. Trends in Pharmacological Sciences, 40(8), 577–591.
Holzinger, A., Biemann, C., Pattichis, C. S., & Kell, D. B. (2017). What do we need to build explainable AI systems for the medical domain? ArXiv Preprint ArXiv:1712.09923. Hummel, P., & Braun, M. (2020). Just data? Solidarity and justice in data-driven medicine.
Life Sciences, Society and Policy, 16(1), 1–18. Lee, E. (2021). How do we build trust in machine learning models? Available at SSRN3822437.
Lip, S., Visweswaran, S., & Padmanabhan, S. (2020). Transforming Clinical Trials with Artificial Intelligence. In Artificial Intelligence (pp. 297–306). Productivity Press. Luengo-Oroz, M., Pham, K. H., Bullock, J., Kirkpatrick, R., Luccioni, A., Rubel, S.,
Wachholz, C., Chakchouk, M., Biggs, P., & Nguyen, T. (2020). Artificial intelligence cooperation to support the global response to COVID-19. Nature Machine Intelligence, 2(6), 295–297.
Maphumulo, W. T., & Bhengu, B. R. (2019). Challenges of quality improvement in the healthcare of South Africa post-apartheid: A critical review. Curationis, 42(1), 1–9.
Mayorga-Ruiz, I., Jiménez-Pastor, A., Fos-Guarinos, B., López-González, R., García-Castro, F., & Alberich-Bayarri, Á. (2019). The Role of AI in Clinical Trials. In Artificial Intelligence in Medical Imaging (pp. 231–243). Springer.
McNeill, P. M., & Walton, M. (2002). Medical harm and the consequences of error for doctors. The Medical Journal of Australia, 176(5), 222–225.
Pavli, A., Theodoridou, M., & Maltezou, H. C. (2021). Post-COVID syndrome: Incidence, clinical spectrum, and challenges for primary healthcare professionals. Archives of Medical Research.
Prabu, A. (2021). SmartScope: An AI-Powered Digital Auscultation Device To Detect Cardiopulmonary Diseases.
Ross, S., Bond, C., Rothnie, H., Thomas, S., & Macleod, M. J. (2009). What is the scale of prescribing errors committed by junior doctors? A systematic review. British Journal of Clinical Pharmacology, 67(6), 629–640.
Shaheen, M. Y. (2021a). Adoption of machine learning for medical diagnosis. Shaheen, M. Y. (2021b). AI in Healthcare: medical and socio-economic benefits and challenges.
Shi, D., Zhang, W., Zhang, W., & Ding, X. (2019). A review on lower limb rehabilitation exoskeleton robots. Chinese Journal of Mechanical Engineering, 32(1), 1–11. Sucharitha, G., & Chary, D. V. (2021). Predicting the effect of Covid-19 by using artificial intelligence: A case study. Materials Today: Proceedings.
Ting, D. S. W., Liu, Y., Burlina, P., Xu, X., Bressler, N. M., & Wong, T. Y. (2018). AI for medical imaging goes deep. Nature Medicine, 24(5), 539–540.
Vaishya, R., Javaid, M., Khan, I. H., & Haleem, A. (2020). Artificial Intelligence (AI) applications for COVID-19 pandemic. Diabetes & Metabolic Syndrome: Clinical Research & Reviews, 14(4), 337–339.
van der Schaar, M., Alaa, A. M., Floto, A., Gimson, A., Scholtes, S., Wood, A., McKinney, E., Jarrett, D., Lio, P., & Ercole, A. (2021). How artificial intelligence and machine learning can help healthcare systems respond to COVID-19. Machine Learning, 110(1), 1–14.
Woo, M. (2019). An AI boost for clinical trials. Nature, 573(7775), S100–S100.
Help us care for others
Join our mailing list to get early updates and early access.